What is Chain of Thought Prompting in AI Reasoning?
Updated on : 23 April, 2025
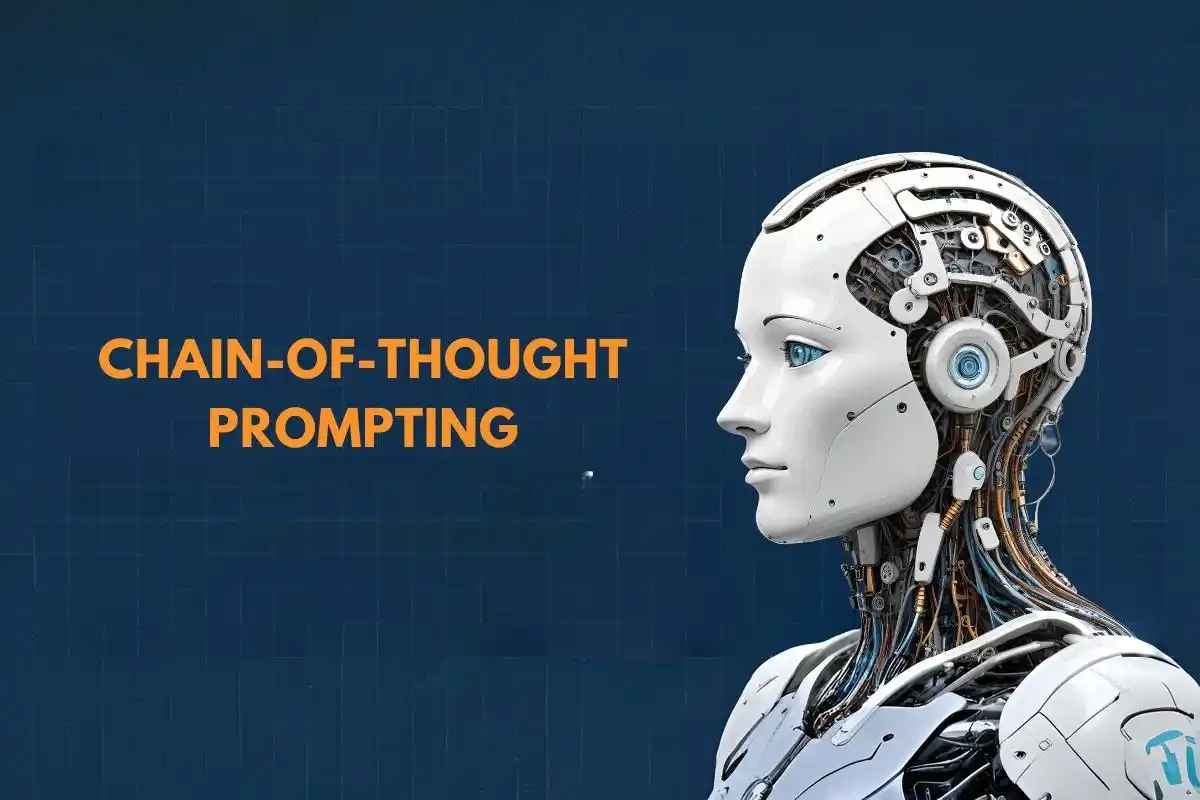
Image Source: google.com
Table Of Contents
- 1. Introduction to Chain of Thought Prompting
- 2. Why Traditional Prompts Fall Short
- 3. What Makes Chain of Thought Prompting Different
- 4. Types of Chain of Thought Prompts
- 5. How It Enhances Reasoning in LLMs
- 6. Real-World Use Cases of CoT
- 7. When Not to Use Chain of Thought Prompting
- 8. Implementation Tips and Tools
- 9. Future of Prompt Engineering with CoT
- 10. How Hexadecimal Software Can Help You Build with Chain of Thought AI
- 11. FAQs
- 12. Conclusion
Table Of Contents
Introduction to Chain of Thought Prompting
Chain of Thought (CoT) prompting is a method used to improve the reasoning capabilities of large language models (LLMs) by breaking tasks down into intermediate steps.
Instead of asking a model for a direct answer, CoT guides the model through a logical path of thinking—just like how a person would explain their reasoning.
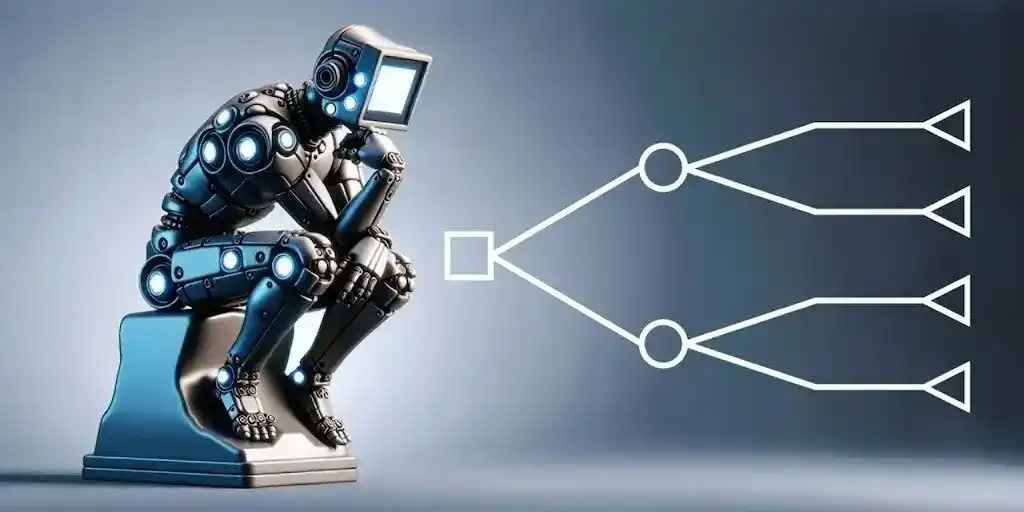
Image Source: google.com
Why Traditional Prompts Fall Short
Most standard prompts aim for quick, direct answers. They’re fine for surface-level tasks like defining terms, converting units, or answering trivia. But when it comes to more complex reasoning, traditional prompting breaks down. Here’s why:
🚫 No Step-by-Step Thinking
Traditional prompts expect the model to jump straight to the answer. This works if the answer is obvious, but in problems requiring multi-step reasoning, the model often skips crucial logic or makes mistakes. Think of it like asking someone for a final answer without letting them use scratch paper.
🔍 Poor Performance on Multi-Hop Tasks
Tasks that require connecting multiple pieces of information—like reading comprehension, legal argumentation, or solving layered math problems—need intermediate reasoning. With a single-shot prompt, there’s no room for the model to break the problem into manageable parts. This leads to shallow or incorrect answers.
🧠 No “Thinking Out Loud”
Humans solve problems by reasoning through them—writing down steps, questioning assumptions, testing ideas. Traditional prompting doesn’t simulate this process. It treats LLMs as calculators, not thinkers. As a result, the model doesn’t "show its work," and errors go undetected.
Prompt Type | Approach | Best For | Weakness |
---|---|---|---|
Traditional Prompting | Ask direct question, expect one-shot answer | Simple facts, definitions, quick responses | Fails on multi-step problems |
Chain of Thought Prompting | Guide model to reason step by step | Math, logic, contextual reasoning | Takes more tokens, slightly slower |
📉 Lower Accuracy in High-Stakes Scenarios
In fields like medicine, law, or advanced finance, even a minor error in reasoning can have serious consequences. Traditional prompting fails to highlight how an answer was derived, making it hard to verify or trust the result.
🔄 No Feedback Loop
Without step-by-step reasoning, there's no feedback loop. You can’t trace where the model went wrong, making it tough to refine prompts or debug logic. With CoT prompting, each step offers a chance to evaluate and guide the model's thinking.
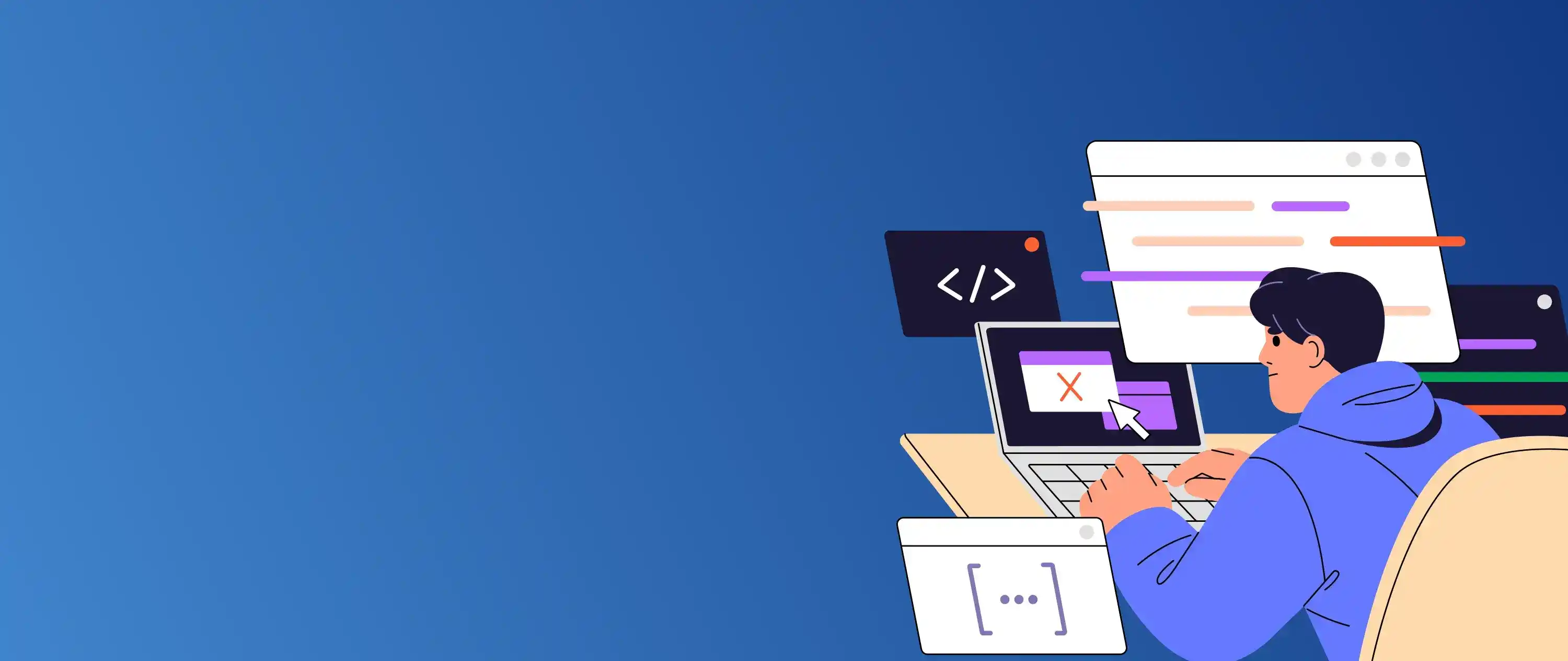
Want to integrate CoT-based reasoning into your product?
What Makes Chain of Thought Prompting Different
Chain of Thought (CoT) prompting works by modeling how humans break complex problems into logical steps. Instead of jumping directly to the answer, the model is encouraged to “think aloud,” outlining the reasoning behind its response.
🧠 Mimicking Human Problem Solving
When we solve problems—especially math, logic, or decision-based questions—we don’t just blurt out an answer. We walk through our thought process, double-check our assumptions, and work through intermediate steps. CoT prompts teach the model to do the same.
Here’s a simple example that illustrates the difference:
Prompt:
“A farmer has 3 cows, each gives 4 liters of milk. How many liters total?”
Traditional Response:
"12"
Chain of Thought Response:
"Each cow gives 4 liters → 3 cows × 4 liters = 12 liters."
By explaining the reasoning, the model offers transparency and allows for better validation and debugging of answers.
Feature | Traditional Prompting | Chain of Thought Prompting |
---|---|---|
Output Style | Direct answer only | Step-by-step explanation |
Use Case | Simple tasks (definitions, trivia) | Complex reasoning (math, logic, analysis) |
Transparency | Low—no explanation | High—explains thought process |
Error Tracing | Hard to diagnose mistakes | Easy to identify missteps |
Build immersive augmented and virtual reality experiences that enhance user engagement, offering innovative solutions for industries like gaming, healthcare, and education.
Types of Chain of Thought Prompts
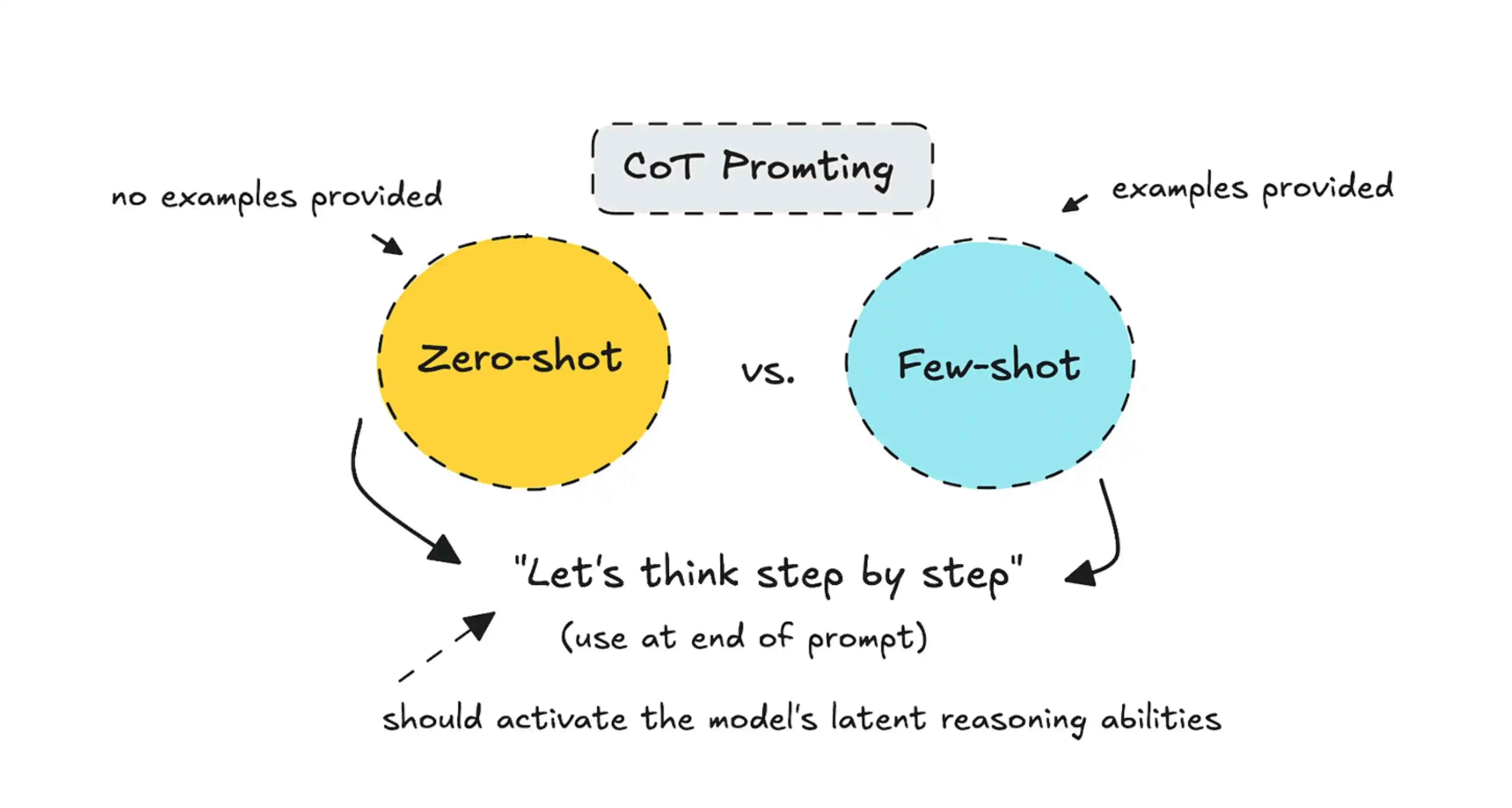
Image Source: google.com
Chain of Thought (CoT) prompting comes in various forms, each suited to different use cases and model capabilities. Whether you’re guiding the model explicitly or nudging it subtly, the goal remains the same: improve reasoning by encouraging step-by-step thinking. Here's a breakdown of the main types:
Prompt Type | Description | Best Use Case |
---|---|---|
Manual CoT | The reasoning steps are written by the user in the prompt. | Math problems, logic puzzles |
Automatic CoT | The model generates the reasoning on its own, without explicit examples. | General question answering, open-domain queries |
Few-shot CoT | Several examples with detailed reasoning are included to guide the model. | Complex decision trees, scientific explanations |
Zero-shot CoT | A single phrase like 'Let's think step by step' cues reasoning without examples. | Quick insights, trivia, lightweight logic tasks |
Key Takeaways:
- Manual CoT gives you the most control but requires effort to craft.
- Few-shot CoT is ideal when you want consistent logic across similar tasks.
- Zero-shot CoT is fast, lightweight, and surprisingly effective for simple logic.
- Choosing the right CoT strategy depends on task complexity, domain, and model size.
How It Enhances Reasoning in LLMs
Chain of Thought prompting doesn’t just improve answers—it fundamentally changes how large language models (LLMs) reason. By nudging the model to slow down and think in steps, CoT reduces guesswork and increases traceability.
Here’s a look at how performance improves with CoT prompting:
Model | Task Type | Accuracy Without CoT | Accuracy With CoT |
---|---|---|---|
GPT-3.5 | Grade-school Math Word Problems | 57% | 82% |
PaLM | Symbolic Logic Tasks | 17% | 53% |
Claude | Multi-hop QA (e.g., reasoning across documents) | 63% | 85% |
Real-World Use Cases of CoT
Chain of Thought prompting isn’t just academic—it’s being used in real tools and products today:
-
📚 EdTech Platforms
CoT helps break down math problems or science questions into digestible steps, mimicking a tutor’s thought process. Tools like Khanmigo (from Khan Academy) use this to enhance digital learning. -
⚖️ Legal Tech
CoT can summarize legal documents while preserving logical structure. It can trace arguments back to source laws or precedents—great for compliance and legal research tools. -
💰 Fintech Applications
Models can walk through financial decisions—such as whether to approve a loan or recommend an investment—by outlining their assumptions and calculations. -
🩺 Healthcare Assistants
For differential diagnosis, CoT helps AI assistants reason through symptoms step by step, narrowing down likely causes before suggesting actions. -
🛠️ Customer Support Bots
Instead of giving vague answers, bots using CoT can walk users through troubleshooting in a structured way, improving problem resolution and customer satisfaction.
These use cases all share one thing in common: they benefit from explainability, multi-step thinking, and trust-building—all strengths of Chain of Thought prompting.
Blockchain Development Services
Develop secure, decentralized applications and smart contracts with cutting-edge blockchain technology to enable transparency, security, and efficiency.
When Not to Use Chain of Thought Prompting
Despite its strengths, CoT prompting isn’t a silver bullet. There are situations where it’s unnecessary—or even counterproductive:
-
⚡ Speed-Sensitive Applications
CoT increases the token count and response time. If latency matters (e.g., voice assistants or real-time systems), a quick answer may be more valuable than an explained one. -
🎯 Ultra-Simple Queries
For questions like “What’s the capital of Germany?” or “Convert 5 feet to meters,” adding reasoning wastes resources without improving quality. -
📏 Strict Output Formatting
Some tasks (like code generation or data extraction) require tight formatting. CoT can introduce variability and verbosity, making post-processing harder.
Use CoT selectively. It shines in complexity—not in trivia. Here are the links in the requested format:
Web Application Development Services
Create dynamic, user-friendly web applications with modern frameworks, ensuring high performance, scalability, and seamless user experience.
Implementation Tips and Tools
Getting started with Chain of Thought prompting doesn’t require special tools—but a few best practices help maximize results:
🛠️ Prompting Techniques
- Use cues like “Let’s solve this step by step” or “First, we...” to trigger reasoning behavior.
- In few-shot CoT, provide 2–3 examples with detailed reasoning steps before the final prompt.
🧰 Model Recommendations
- OpenAI GPT-4: High accuracy and great at multi-step logic
- Anthropic Claude: Tuned for structured reasoning and safety
- Google PaLM 2: Strong performance on reasoning-intensive benchmarks
📚 Helpful Tools
- PromptSource: A community-driven repo of prebuilt prompts.
- LangChain + CoT: Combine reasoning with tools like search APIs or calculators.
- Notebook-based prototyping: Try Google Colab or Jupyter to iterate on prompts and observe output behavior.
For even better performance, combine CoT with tool usage—like letting the model use a calculator or call an API to verify steps in real time.
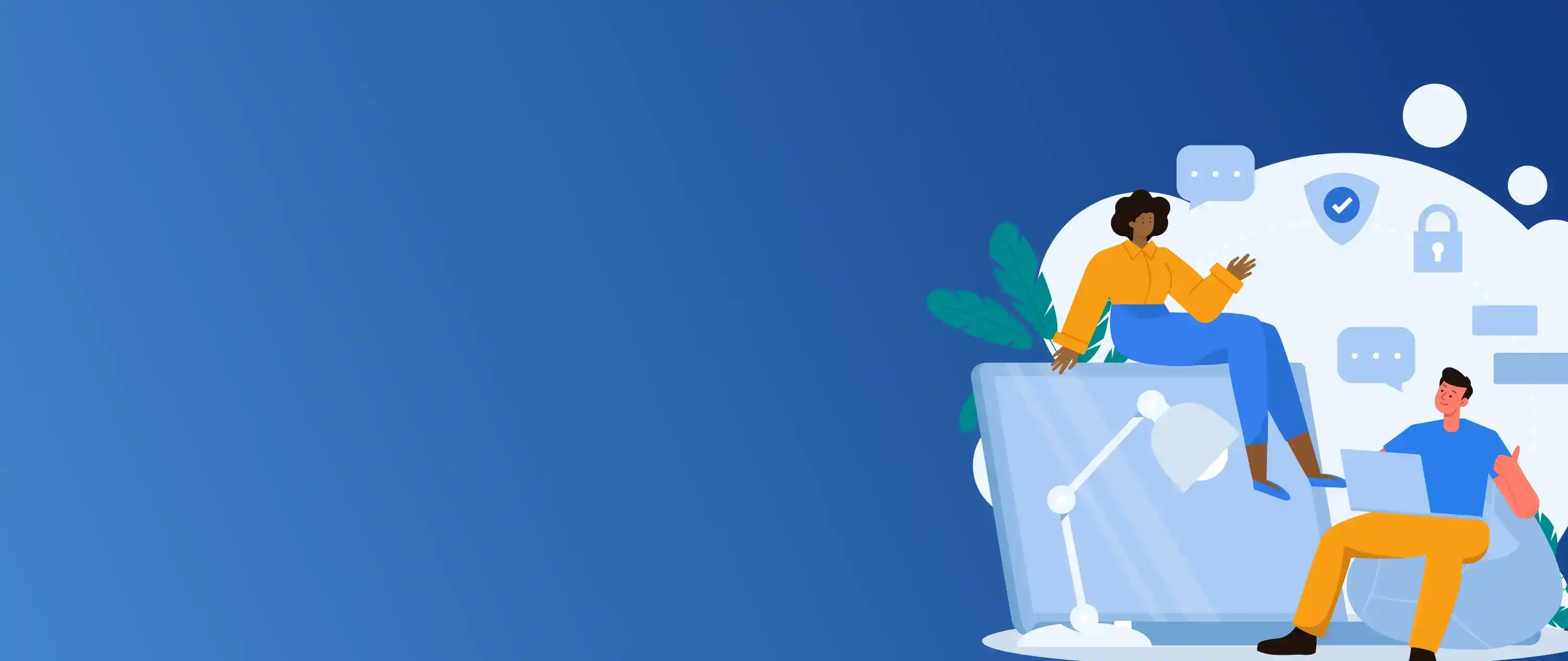
Build smarter AI with Chain of Thought prompting and explainable reasoning.
Future of Prompt Engineering with CoT
As LLMs evolve, Chain of Thought prompting will become central to how we build and trust AI systems. Expect to see it integrated into:
🤖 AutoGPT and Multi-Agent Systems
CoT will serve as the reasoning core for AI agents—letting them plan, self-correct, and reflect across multiple steps before acting.
🧩 Multi-Modal LLMs
With models that process images, speech, and text, CoT will allow them to combine visual and textual reasoning. Think: analyzing a chart and explaining it in steps.
📘 Pretrained CoT-Capable Models
Future LLMs may be fine-tuned to default to Chain of Thought reasoning, reducing the need for engineered prompts. They'll naturally "think before they speak."
🧠 CoT + External Memory
Imagine models that not only reason in steps, but also log and recall previous reasoning—creating coherent thought chains across interactions, sessions, or agents.
CoT isn’t just a clever hack—it’s becoming core infrastructure for explainable, powerful AI reasoning.
How Hexadecimal Software Can Help You Build with Chain of Thought AI
At Hexadecimal Software, we work with startups, enterprises, and product teams to build intelligent, explainable, and secure AI systems—leveraging Chain of Thought prompting, reasoning models, and LLM integrations to deliver smarter digital experiences.
🧠 CoT-Powered Application Development
We design AI features that think like humans—step-by-step. Whether it’s tutoring, financial reasoning, or multi-hop Q&A, we build workflows that reflect how users solve problems.
🔧 End-to-End LLM Integration
From model selection to prompt design and deployment, we integrate GPT-4, Claude, PaLM, or open-source LLMs into your product using scalable architectures and thoughtful reasoning flows.
🔍 Explainability by Design
We prioritize transparency and traceability. CoT allows users to see how AI reached a decision—ideal for finance, healthcare, legal, and other high-stakes domains.
🔗 Tool-Augmented Reasoning
We combine CoT with calculators, search APIs, data stores, and external tools so your AI can reason and act. Think of it as decision intelligence for real-world tasks.
🔐 Security & Compliance
All our AI builds come with enterprise-grade protections: data encryption, audit logs, GDPR-ready flows, and best practices for model safety and reliability.
⚙️ Continuous Optimization & Monitoring
We don’t stop at launch. We monitor performance, gather user feedback, and refine prompts, memory handling, and reasoning depth based on real usage.
🚀 Connect With Us
Build AI features that reason step by step—just like your users do. From onboarding to decision-making, we help you create smarter, more transparent experiences that users can trust and understand.
FAQs
Q1: Do I need a large model to use Chain of Thought Prompting?
A: CoT works best with models like GPT-3.5 or larger, but can be adapted for smaller models with fine-tuning.
Q2: Can I automate CoT prompt creation?
A: Yes, using prompt generation libraries or templating tools.
Q3: Is Chain of Thought only useful for text-based tasks?
A: It’s most effective for text, but multi-modal CoT is an emerging area.
Q4: Can you build a custom CoT-based AI assistant for my business?
A: Absolutely. We specialize in custom AI solutions across domains.
Q5: Is Chain of Thought the same as multi-step reasoning?
A: Similar, but CoT emphasizes explainability and transparency in those steps.
Here are some additional, high-quality FAQs to expand your section and address a broader range of reader concerns—especially around implementation, performance, and business use cases:
Q6: Will using CoT increase token usage and cost?
A: Yes, CoT generates longer outputs, which can slightly increase token count and API costs. But the trade-off is better reasoning and higher answer accuracy—critical in high-value applications.
Q7: Can I use CoT prompting in production environments?
A: Definitely. Many companies use CoT in customer support bots, finance tools, and tutoring systems. We help optimize prompts and architecture for reliability and speed.
Q8: Do I need fine-tuning to use CoT effectively?
A: Not necessarily. You can use CoT effectively with zero-shot or few-shot techniques. Fine-tuning can improve performance but isn’t required for many use cases.
Q9: Can CoT be used with Retrieval-Augmented Generation (RAG)?
A: Yes. CoT enhances RAG by helping the model reason through retrieved information step by step, improving context handling and factual accuracy.
Q10: What’s the difference between CoT and prompting with examples?
A: Examples (few-shot) show the model what a good response looks like. CoT is about structuring the model’s internal reasoning. They work well together.
conclusion
Chain of Thought prompting is a game-changer for AI reasoning. By guiding models to think step-by-step, it enhances accuracy, transparency, and trustworthiness. As AI continues to evolve, CoT will play a crucial role in shaping how we interact with intelligent systems.